Abstract
Robotic tactile perception is an important enabler of precise and robust manipulation in uncertain, cluttered, and/or vision-limited environments. However, there is no standardized tactile sensor used for robotic applications, which creates the need to develop new algorithms for similar tasks depending on the tactile sensor available. To address this challenge, we propose tactile cross-modal transfer — a method of rendering a tactile signature collected by one sensor (“the source”) as though it is perceived by another sensor (“the target”). This method enables us to deploy algorithms designed for the target sensor seamlessly and without modification on signatures collected by “sources”. We implement this idea using several generative models for cross-modal transfer between the popular GelSlim 3.0 and Soft Bubble tactile sensors. As a downstream task, we test the method on in-hand object pose estimation from Soft Bubble but using GelSlim images as input. This task demonstrates the transferability of tactile sensing as a building block toward more complex tasks.
Method
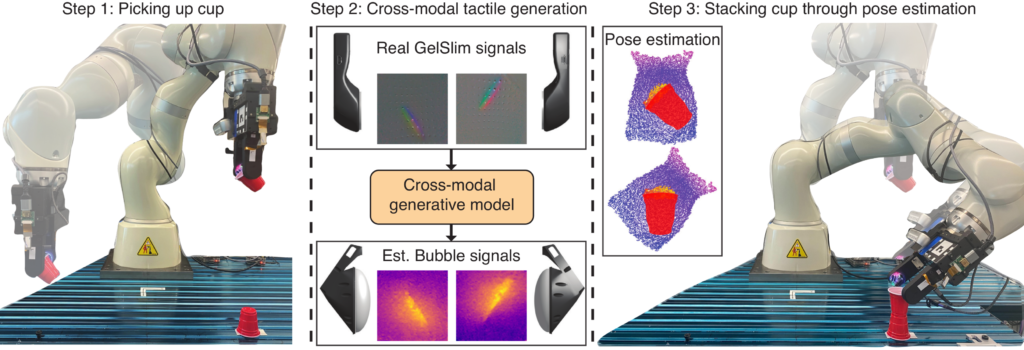
Experiments
Project Details
Datasets, Methods Implementation, Robotics Task Details
Datasets
Sensor Alignment
For our Touch2Touch dataset, we align both sensors by locating the grasp frame of each at the same section of the manipulated object to obtain paired tactile signatures. In addition, we keep both sensors at a distance D. The final step to align the tactile signatures is to rotate one sensor image by 180◦. This rotation is necessary for the grasp frames to be aligned. We can see the grasp frames projection in the image plane of each sensor and notice the need to rotate for alignment. This figure also shows the difference in size between the sensors’ images in pixel space and millimeters.
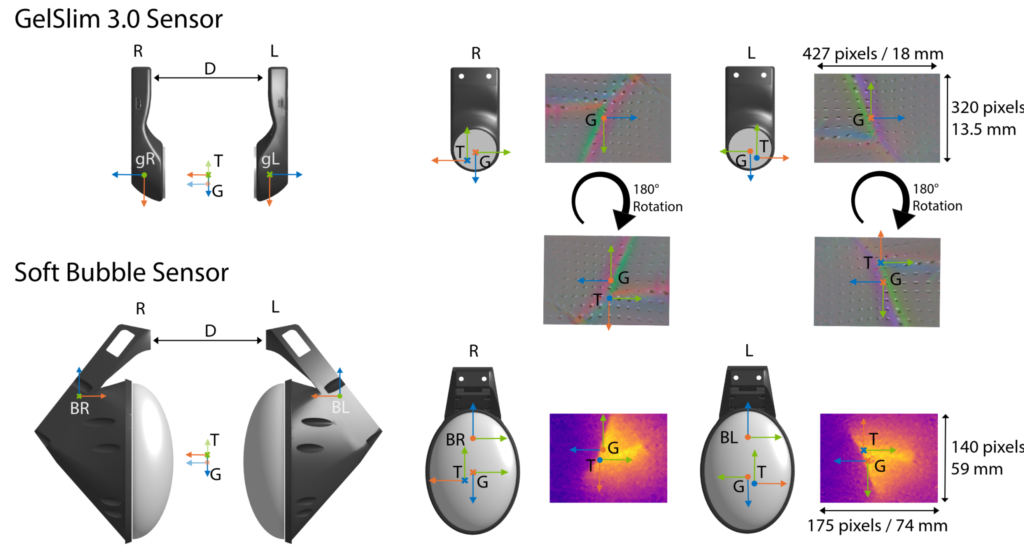
Unambiguous Dataset
We obtain paired tactile signals for 12 different tools. The touch samples were collected within a 10mm x 10mm grid centered at the tool origin, with sensor angles in the range ±22.5◦. We collected 2,688 paired samples per tool for a total of approximately 32,256 paired samples. Details about the dataset composition are shown in the table below.
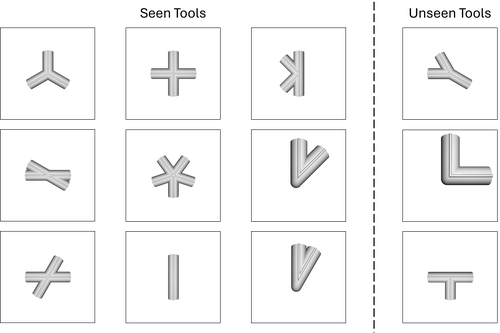
Dataset Split | Tool Set | # of Tools | Sample % | Purpose |
Training | Seen | 9 | 80 | Training |
Unseen Grasps – Validation | Seen | 9 | 10 | Tuning |
Unseen Grasps – Testing | Seen | 9 | 10 | Testing |
Unseen Tools – Validation | Unseen | 3 | 10 | Tuning |
Unseen Tools – Testing | Unseen | 3 | 10 | Testing |
Ambiguous Dataset
We obtained paired tactile signals for 18 different tools: 15 were used for training and 3 for testing. The touch samples were collected within a 10 x 10 mm grid around the tool center and at different sensor angles with respect to the tools in the range [-22.5◦, 22.5◦]. We collected paired samples per tool for a total of approximately 8.3K paired samples. During this data collection procedure, we do not restrict the data collection to only samples that contain key features of the tools, which makes the dataset ambiguous.
Methods Implementation
Diffusion Model
Our implementation of the diffusion model closely follows Stable Diffusion, with the difference that we use a ResNet-50 to generate the GelSlim encoding from GelSlim images for conditioning. The model is optimized for 30 epochs by Adam optimizer with a base learning rate of 10-5. The learning rate is scaled by gpu numberxbatch size. We train the model with batch size of 48 on 4 NVIDIA A40 GPUs. At inference time, the model conducts 200 steps of the denoising process with a 2.54 guidance scale.
VQ-VAE
We use a VQ-VAE architecture similar to the one proposed by Van den Oord et al for the style transfer. Before training VQ-VAE, we processed the sensor images by obtaining the difference between the deformed image at the moment of contact with the object and the undeformed image. In addition, we resize these images from the sensor images’ original size to 128×128 and keep their corresponding numbers of channels. The input to our model is a 128×128 subtracted Gelslim RGB image, and the output is the corresponding 128×128 subtracted depth map Soft Bubbles image. The input image x is passed through a CNN encoder to generate a vector in the latent space z. This latent vector is then quantized via a collection of discrete vectors known as the codebook, such that ze(x) is transformed into zq(x). This quantized latent vector is passed through a CNN decoder to generate the final image x̄. The encoder parameters, quantization codebook vectors, and decoder parameters are all learned such that mean squared-error in the latent space quantizations and output reconstructions are minimized.
L1 & VisGel
We use the VisGel model proposed by Li et. as the first baseline method, which trains a conditional GAN model for tactile signal generation. The L1 model follows exactly the same architecture as VisGel but is trained to only minimize an L1 loss.
Robotics Task Details
One of the ways that we evaluate our cross-modal touch prediction model is by estimating object pose from generated touch signals and using this pose to accomplish downstream tasks.
In-Hand Pose Estimation
To obtain object pose, we align the generated and measured Soft Bubble point clouds to their corresponding object geometry point clouds using ICP, which finds a rigid transformation between the two. To perform ICP, we first need to obtain points belonging to the object surface from the full Soft Bubble point cloud. We obtain these points by training a UNet ~\cite{unet} to generate a mask from the raw Soft Bubble images. To acquire our second point cloud for ICP, we sample object surface points from a CAD model. The CAD model is aligned to the grasp frame of the sensor. We apply ICP to these point clouds to find the transformation that aligns the two and provides the relative pose of the Soft Bubble sensor with respect to the object. Using the inverse of this transformation, we obtain the in-hand pose estimate of the object with respect to the grasp frame of the sensor.
Soft Bubbles Masking
To obtain the contact patch mask: (1) we get the contact points between the Soft Bubbles point clouds and the tool by using Signed Distance Function (SDF) and preserving only the points that are close to the surface, (2) we transform these points to the image space to create the mask, and (3) we train the UNET on the Soft Bubble portion of our dataset with the corresponding masks.
Peg-in-Hole Insertion
The peg-in-hole insertion task consists of several steps: (1) we hand an object to the robot in a random orientation, (2) the robot grasps the object with GelSlim sensors, (3) we apply our cross-modal tactile generation model online to obtain a predicted Soft Bubble signal, (4) we perform ICP on the predicted Soft Bubble signal to estimate the angle of the object with respect to the grasp frame of the GelSlim sensor, (5) we align the object with the insertion hole using the estimated angle, and (6) we insert the object in the hole. We evaluate this task on three tools that were unseen during training and one real object (a pencil).
Cup Stacking
The stacking task utilizes a procedure similar to the insertion task. The main differences are the success criteria and the object geometry. The stacking task is completed successfully if one small SOLO-brand cup is balanced on top of another.