As humans, we are able to seamlessly integrate our senses of sight and touch to learn about our physical world. These two modalities provide complementary information, for where sight provides global but coarse information, touch provides dense and highly discriminative but very local information. Not only do we see and feel our world, but we also categorize and build useful abstractions to facilitate our manipulation skills. For example, when interacting with a door, we may infer it is locked or open (two useful abstractions we have constructed) through how it feels and moves.
We are interested in how robots can acquire similar abstractions and concepts through physical interaction. For example, how can a robot learn useful abstractions and physics models in the joint domain of touch and touch to play Jenga. Inferring useful abstractions such as blocks that do and don’t move helps the robot to quickly give up on risky interactions or to continue pushing to moveable block. We have demonstrated one approach to this problem (Science Robotics 2020); however, this is an exciting and rich space to explore.
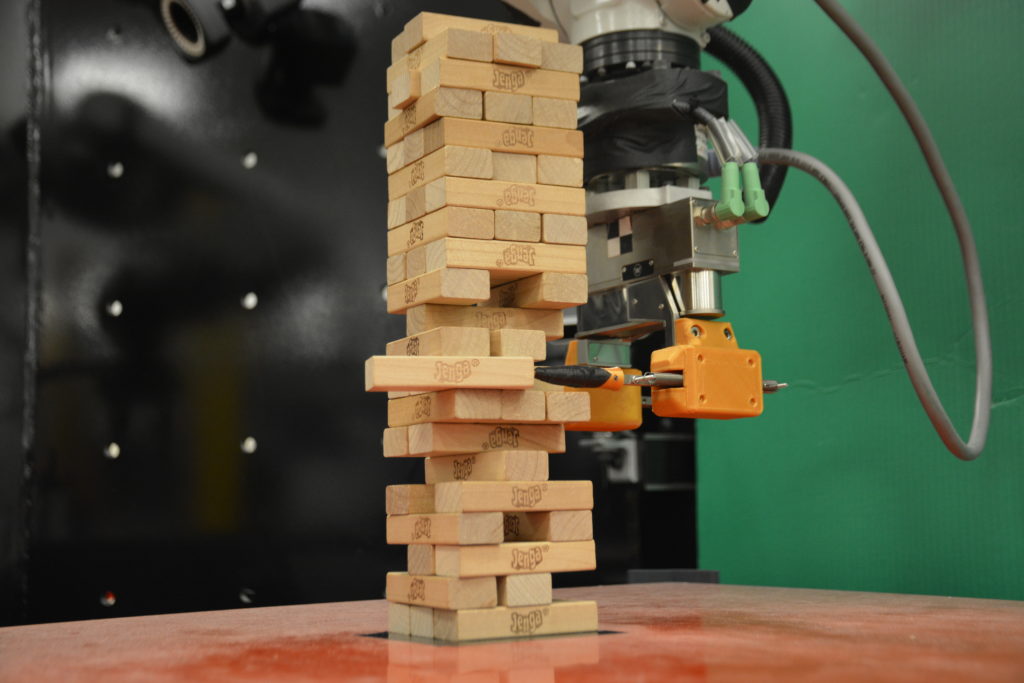